My interest in digital marketing started in my university years. I studied ‘Management Engineering’ degree, which had a curriculum including both business classes as well as advanced math, statistics, economy etc. Compared to other Business Administration degrees, Management Engineering graduates are more analytical people with a deep understanding of various business disciplines such as finance, marketing, operations etc.
Since those years, I had two passions: math and marketing. Eventually, I became ‘a nerd marketer’, which is why performance marketing has been a sweet spot for me to build my career on, and get deeper on this specific branch of marketing. Among all other definitions, I like this definition of performance marketing I found on Linkedin1:
#performancemarketing is a data-driven approach that emphasizes driving specific actions, such as conversions, sales, or leads. Unlike traditional marketing strategies that focus on broad brand awareness, Performance Marketing is centred around measurable outcomes and ROI. It relies heavily on key performance indicators (KPIs) and analytics to optimize campaigns and allocate resources effectively.
Measurement in Performance Marketing
As highlighted above, ‘data-driven approach’, meaning ‘measurement’ is at the heart of performance marketing. Thanks to Google, which has been offering Google Analytics for free for over a decade, measurement of online sales, leads, subscriptions (and basically any conversion) is accessible to basically everyone on the planet. They made it very easy for people to implement a few lines of codes to access fundamental measurement capabilities.
Almost all e-commerce companies primarily used Google Analytics to track the visitor activity on their websites and made key decisions on their design and user experience as well as marketing campaigns, such as Google Adwords, obviously. Google made it incredibly easy for e-commerce companies (and other performance marketers) to use Google Adwords to promote their products, use Google Analytics to measure the success of these campaigns, and optimize campaign creative, keyword, targeting. This perfect synergy has been at the core of what made Google an advertising behemoth.
What is an ‘Attribution Model’?
Everyone sees, and sometimes interacts with ads on websites and mobile apps, be it on Instagram, or Google, or Wall Street Journal. When people interact with the ads either by viewing (e.g video ads on Youtube), or clicking, they create a ‘touchpoint’ for the advertiser. Advertisers need to understand the impact of each ‘touchpoint’ on the intention of the user taking a particular action, such as purchasing their product. This helps advertisers allocate their limited advertising budget more effectively across different advertising platforms (aka publishers) for maximum outcome – meaning higher Return on Investment (ROI), more accurately, Return on Ad Spend (ROAS).
Therefore, advertisers needed to use certain ways to ‘attribute’ values to each touchpoint before a conversion. Thus, attribution models were born.
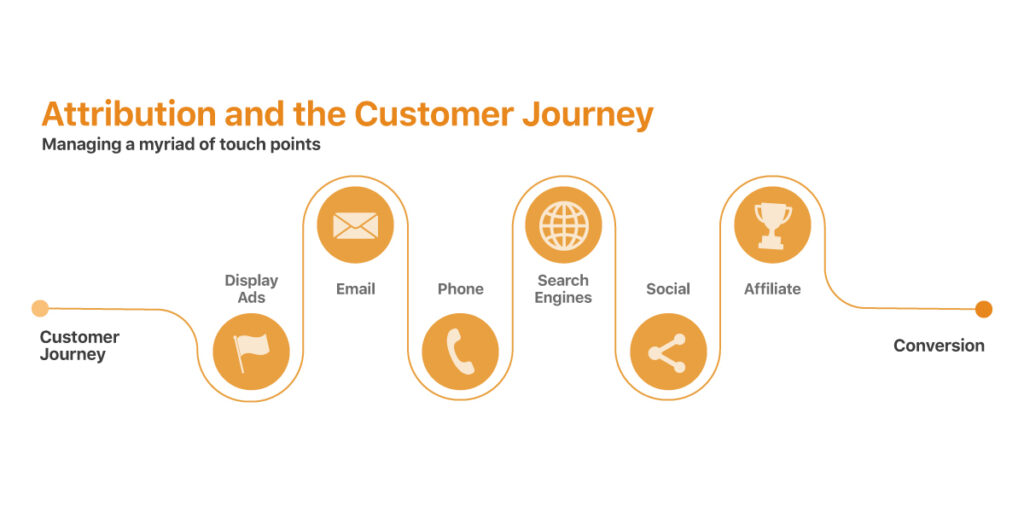
How to Choose the Best Marketing Attribution Model – Adparlor
Last-click attribution, which means attributing the entire value of the conversion to the last click of the user before conversion, became a global standard in any digital marketer’s decision making process on measurement tools like Google Analytics. It was a powerful way to measure success, and delivered great results in the early days of the digital advertising.
However, not long after, digital marketers realized that last-click attribution was not the most effective way to evaluate the value of each marketing channel. It was overvaluing advertising platforms at lower points of the funnel reaching people with higher intent (e.g Google), and it was undervaluing the advertising platforms at higher points of the funnel reaching people with lower intent (e.g Meta, display networks etc). Therefore, advertisers started to move away from last-click attribution towards ‘multi-touch attribution’ models, which meant each touchpoint of the user before converting was given a certain value – not only the last click. The weight of the value was determined based on the model chosen by the marketer. There are a few popular models like U-shaped (value the last and first interaction the most), time decay (value decreases from last to first touchpoint) etc. On top of them, some measurement companies also offer more advanced, statistically changing attribution models for more effective attribution logics (as opposed to static models shown below).
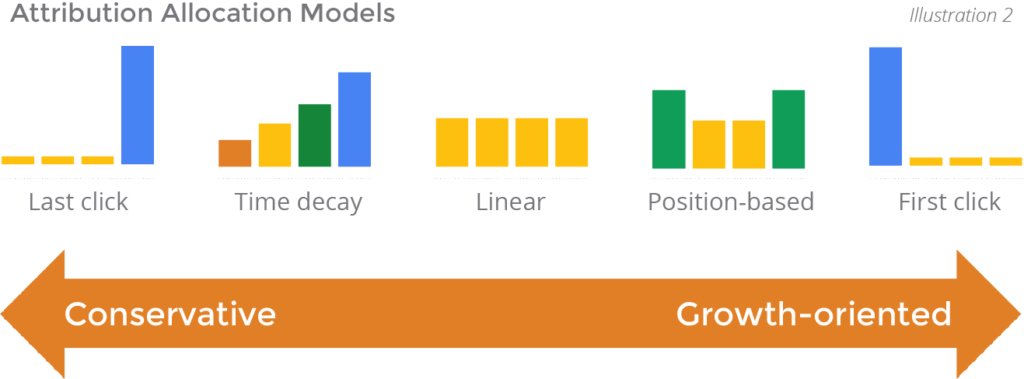
An In-Depth Look at Attribution Modeling in Digital Marketing (Humanlytics)
At the end of the day, marketers experimented with different models, and decided on the one which predicted the value of each channel the best, leading the highest ROAS for their digital advertising budgets.
But, how does it technically work?
Attribution measurement solutions are based on ‘proxy’ technologies, such as third party cookies (in short cookies) on web browsers, or identifiers for mobile apps. This is how cookies works:
- Website implements the analytics tools’ (e.g Google Analytics) piece of javascript code on all pages
- This javascript code is run whenever any of the website’s page is viewed by any user on a browser (Safari, Chrome etc).
- This code uses the ‘cookies’ in the browser to inform the analytics tool about a particular user’s activity (e.g viewing a product, adding a product to cart, subscribing to a newsletter etc).
- Analytics tool aggregates all the data and provides insights and measurement to the website.
With the increasing popularity of smartphones and the widespread preference for mobile apps over mobile websites, new technologies were introduced in mobile operating systems to cater to these trends. For mobile apps, traditional cookies made way for IDFAs on iOS devices and AAIDs (also known as Google Advertising ID) on Android devices. Alongside these advancements, new attribution tools known as Mobile Measurement Partners (MMPs) emerged to effectively track activities within mobile apps, (eg. games), utilizing these technologies.
Impact of iOS14 changes
iOS14, specifically iOS14.5, was a major milestone in digital advertising. Why? Because Apple announced an important change on how users can be tracked in iOS for advertising purposes.
“App Tracking Transparency requires apps to get the user’s permission before tracking their data across apps or websites owned by other companies for advertising, or sharing their data with data brokers. Apps can prompt users for permission, and in Settings, users will be able to see which apps have requested permission to track so they can make changes to their choice at any time.” Apple’s official announcement of iOS14.52
This meant that users were going to start seeing this prompt first time they open any app after these changes:
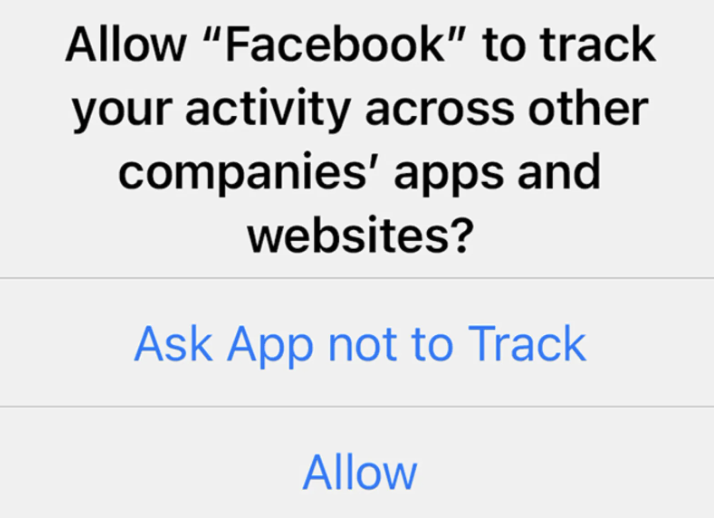
And most people chose the “Ask app not to track”, unsurprisingly. This meant, for majority of iOS users, apps could not do any realtime IDFA-based measurement or targeting anymore.
Side note: This was supposed to be a good thing for the user. However, I personally think that most users did not understand what exactly this prompt meant. It would be unfair to expect users to truly understand the pros and cons of choosing either option (e.g not allowing simply meant irrelevant ads), and make an informed decision, which would be ideal. I also understand that users are extra sensitive about ‘tracking’, and ‘personal data’, so their default has been a solid no for almost all iOS apps.
Apple, with these changes, opened a new world for digital advertising, and led to important paradigm shifts. They forced advertisers to seek alternative ways to measure success of their digital advertising investments.
For cookies, there has been less strict but a similar process, primarily led by GDPR3 requirements from European Union, enforcing all websites to explicitly ask their visitors to decide whether or not they give ‘cookie tracking’ permissions.
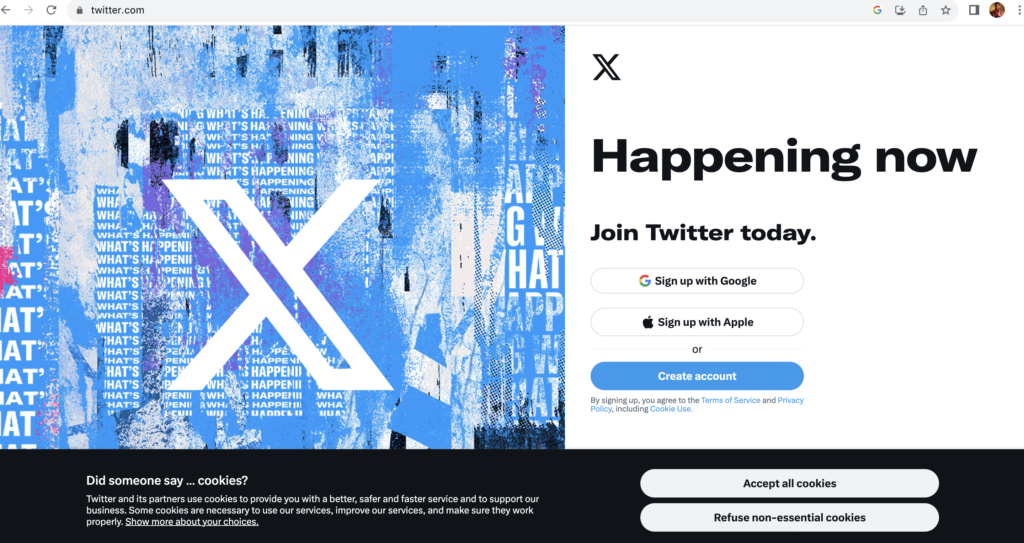
These prompts are less aggressive compared to iOS, and have more friendly wording
Similarly, Google will deprecate third party cookies on Chrome as well as Advertising IDs on Android. They are explaining the process, technology and the new way of doing measurement and targeting on this website: www.privacysandbox.com
After all, with all these major changes, the measurement technologies must adapt, and evolve the way digital advertising being measured. Precisely because of this, a few measurement methodologies have been on the rise, such as: Data Clean Rooms and Media Mix Modeling (MMMs). Neither of these measurement methods are ‘new’ but they are now on demand much more than ever before due to their resilience against these changes. While I plan to do further deep dive about both methodologies in the future, lets do a quick intro on them below.
Data Clean Rooms
What it is:
In a data clean room environment, two parties can securely share and analyze data with full control of how, where, and when that data can be used.4
These two parties can be a retailer and a brand, or an advertiser and a publisher, or any two companies who have major overlap in their audiences and need to analyze their data with the other party’s for valuable insights. It is critical to understand that these environments are designed to ensure that each party does not have access to the other’s sensitive data (e.g Personally Identifiable Information – PII). These solutions provide audience matching technologies with advanced encryption to maximize the security of each party’s data while providing different services such as aggregated measurement and expanded targeting capabilities.
Data Clean Rooms has become particularly very popular in the last few years. Google and Amazon already provide data clean room services through their cloud infrastructures5, though, being a neutral third party with deep experience on data processing, Liveramp has been one of the leading service providers in this space.
Media Mix Modelling (MMM)
MMMs, also known as Marketing Mix Models, have been in use for several decades, primarily favored by brand advertisers owing to their capacity to measure the impact of marketing campaigns on sales across all channels.
How it works:
Quoting from Marketing Evolution:
“…MMM uses aggregate data;As such, it is able to evaluate a wider range of channels, both traditional and digital. Additionally, MMM allows marketers to factor in external influencers such as seasonality, promotions, etc. …The statistical analysis performed by media mix modeling uses multi-linear regression to determine the relationship between the dependent variable, such as sales or engagements, and the independent variables, such as ad spend across channels. For example, MMM can use both linear and non-linear regression methods to determine how increased marketing spend on magazine ads affected overall sales. To get the most robust and accurate visibility into marketing impact, several models should be evaluated.”
The two highlights are important; aggregate data and multi-linear regression. Aggregate data means MMM does not require PII or proxy-level data for measurement unlike other methods, which means MMM is resilient against changes to cookies or identifiers. Multi-linear regression means it includes statistical modelling which makes it more complex but also open for constant improvements unlike static attribution models used historically by marketers.
But, MMM also comes with caveats. They were historically run by companies like Nielsen, IRI etc, who receive aggregated sales data from retailers and map them to the brand’s advertising data to do their analysis. These MMM studies were expensive, and take long (3-6 months) to execute and produce results. They provided macro level insights on effectiveness of particular advertising channel to which help brands/advertisers make media mix decisions but they fall short on providing more tactical recommendations on how to improve media mix or campaign setups on a regular and frequent basis.
However lately, there are now more open source MMM offerings and startups, which are cheaper alternatives, and aim to make the analysis more real-time and actionable. Even Amazon today offers self-serve MMM measurement to advertisers in select countries6
Important to note that while all publishers such as Google, Meta, Amazon provide different self-serve measurement solutions (attribution, data clean rooms or MMM), advertisers tend to prefer third-party neutral companies like Neustar, Nielsen, IRI to avoid any conflict of interest.
All in all, Apple and European Union, which will be followed by Google, with their stance on the data privacy regulations, kicked off major changes in digital advertising. Now, advertisers are actively listening to the publishers and measurement companies on how to adapt to this paradigm shift with possibly new ways of measuring success of their digital advertising efforts. While these technologies are still evolving, I think they will significantly increase effectiveness of digital advertising over time without compromising the privacy of users.
References;
- Performance Marketing vs. Digital Marketing: Understanding the Key Differences and Synergies by Anubhav Tyagi
- iOS 14.5 delivers Unlock iPhone with Apple Watch, more diverse Siri voice options, and new privacy controls by Apple
- Complete guide to GDPR compliance
- It’s time to come clean – the complete data clean rooms guide by Appsflyer
- Secure and privacy-centric sharing with data clean rooms in BigQuery by Google & AWS clean rooms by Amazon
- Introduction to Self-Service Marketing Mix Model (MMM) Onboarding by Amazon Ads